Over the next two weeks, Headlight is publishing a three-part series breaking down one of the ways we’re incorporating emergent AI technology to solve problems and improve marketing efficiency across our org. Large Language Models have been transformative in our ability to rapidly process and analyze large swaths of text. In Part 1: The Problem, we outlined how we’re leveraging this new tool to help solve a core competitive intelligence need for our client partners.
In Part 2: The Process below, we walk through our tactical process step-by-step, illustrating how we transform large swaths of previously unactionable qualitative data into a set of fresh consumer sentiment themes that will inform critical marketing and product strategy decisions. Next week, in Part 3: The Pitfalls, we will spell out a variety of tips and tricks we’ve learned through many iterations of this process that will help you ensure your data is accurate and impactful. Subscribe to our blog to stay informed.
“Knowledge has to be improved, challenged, and increased constantly, or it vanishes.” ~Peter Drucker
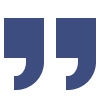
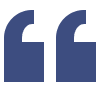
How to Use ChatGPT to Analyze Customer Reviews
“Spilling the T.E.A.”
Headlight has been experimenting with AI and integrating AI into our workflows. One of the innovations, is Headlight has developed an AI-powered process to analyze customer reviews. Internally we call this process T.E.A. — short for Thematic Evaluation Algorithm — to “read the tea leaves” and extract customer insights. At its core, this is similar to a SWOT analysis where we compare our brand with our competitive set to identify key strengths, weaknesses, opportunities, and threats.
We use a much more robust version for our client partners, though the essentials are broadly outlined below with a sample analysis of the “DraftKings” app.
The Process
1. Data Gathering
Gather and consolidate your data set from the App Store, Google Play, or Amazon. If you use a centralized review platform like TrustPilot or Yotpo this will be much, much easier. We have found sorting by “Most Recent” is most useful since we want our insights to be as fresh as possible.
We recommend keeping reviews segmented by platform source as it’s very common that different devices attract different customer types who will have distinct opinions and experiences. What if technical issues are only impacting a recent build of the Android app? Alternatively what if new iOS changes introduced new expectations around privacy? What if different markets have different nuances? For multinational brands segmenting by country or language can also help identify regional differences that would otherwise be difficult to discern. Again, the currency of LLMs is language so “T.E.A.”-sing out the regional differences is most definitely in their wheelhouse.
2. Structure Your Data
Organize your review data with the Source, Reviewer, Review Source, Rating, Review, Version, etc. Depending on your technical proficiency this can take different forms. For example, a JSON file if pulled via API such as iOS App Store Connect or in a spreadsheet for everyman business users. In our example, we have App Store reviews that we’ve converted into a structured text format so we can easily verify ChatGPT’s outputs.
3. Import Files to ChatGPT
Prepare a single doc for quick analyses, or upload up to 10 files in ChatGPT. To do so, simply drag and drop the files or click on the paperclip icon to attach your files with your initial prompt.
Keep in mind that there is a hard limit of 512MB per file at the time of publication. In practical terms, we have tested analyzing a sample of up to 500 reviews very easily. However, file limits is an area OpenAI has begun making changes so this may be a moving target and these limits are subject to change. It’s best to consult directly with OpenAI’s latest documentation or platform updates for the most current information.
4. Test Prompt
After uploading, run your first test prompt to verify the number of reviews you uploaded and enforce that ChatGPT must analyze all reviews. Double check your results manually against your backup file to validate ChatGPT is following instructions.
This is a key step as this question is a closed question with a clear black and white correct and incorrect answer. If ChatGPT gives an incorrect number, you know how much sampling is occurring. The vast majority of issues in our testing have to do with ChatGPT being “lazy” by sampling data to conserve processing power.
5. Strengths and Weaknesses
Now for the more substantive information. Ask what the main themes, strengths, weaknesses or pain points of the app or product are using all the reviews.
6. Back Up with Customer Quotes
Reprompt to verify with direct sourcing of relevant customer quotes, author names, rating and review date so it can be cross-referenced. You could also run additional steps to check the prevalence of these sentiments, or dive deeper into certain themes.
7. Pain points and Weaknesses
Isolate specific weaknesses within your own product or app as opportunities to improve. What are some specific offers marketing can design and promote to address different customer subsets? What are some specific weaknesses compared to competitor’s products or apps?
Even when asking for direct quotes mistakes can happen, so make sure to cross-reference and verify. Here in an earlier test we see ChatGPT flags some Signup issues that could impact acquisition efforts. However, ChatGPT has incorrectly flagged this review that has a positive sentiment with the ease of betting as an issue because of complexity of other platforms. So the picture is more a mixed bag on this point.
8. Opportunities and Threats
Identify surprising needs that customers recommend. Are there new popular feature opportunities? In some cases negative reviews also reveal threats from alternatives to your product.
9. Turn into Something Usable
Turn the Strengths into specific Selling Points you can use in creative or copy. What are the best ways to use these advantages?
In our sample here timeliness of betting is a key factor that can be isolated and framed to take advantage of key opportunities in real-time.
In Headlight’s testing, we have identified cases where subsets of customers require a different message or offer. One of our favorite cases is to identify unique selling points (USPs) or reframing of problems for creative concepting. We then use human wisdom and expertise to prioritize those ideas for maximum impact based on other known performance or priors. If we prefer, we can also identify “blue ocean” strategies of untapped potential the market is missing. A key part is providing the proper context for success and embracing constraints can help guide the AI model or even marketing teams to find otherwise inaccessible solutions.
PS: Part 3 of this series will walk you through the biggest do’s and don’ts when using ChatGPT and LLMs to analyze customer reviews as we learned through iterations developing Headlight’s T.E.A. process. We will share the biggest pitfalls and key examples which are perhaps the most intriguing part and will be released in the next week. Subscribe to stay informed on Part 3 about how to incorporate this strategy and other trends relevant to your business.