Quick Read
- The current state of digital marketing measurement is very much in-flux; app marketing has been impacted most of all.
- Given the accelerated rate of change across platforms, operating systems, and measurement partners, keeping up is nearly impossible.
- We wanted to give a thorough but digestible summary of where things stand, and what your options are as-of publishing time in late July 2024.
- For all of the frameworks discussed below, a good Mobile Measurement Partner will help you bridge the various technical hurdles and provide the most detailed data possible.
- To truly maximize marketing effectiveness, it’s essential to adopt a holistic approach that blends the strengths of various attribution methods, complemented by advanced analytical techniques and direct consumer feedback.
The Context
The rapidly evolving and increasingly fractured state of digital marketing measurement is one of the most discussed topics across the growth marketing ecosystem in 2024. From the resurgence of Media Mix Models (MMM), to the sustained prominence of fingerprinting, to the endlessly delayed (and now abandoned!) sunsetting of third-party cookies, there are many important moving parts that can have systemic impact on advertisers’ ability to drive channels and evaluate performance.
One area that we at Headlight feel has gone under-discussed and, based on the various conversations we participate in, could benefit from further education, is the current suite of attribution systems supporting the mobile app space. What are each of them used for? How does this vary by advertising channel and operating system? And, most critically, how does one tie them together to provide a comprehensive, “truthful”, and actionable set of numbers that builds confidence and drives strategic decision-making?
In this article we will summarize the current state of the app attribution landscape in 2024 – what are the differences in data sources, how and when do you apply each of them, and what are the critical blind spots to be aware of.
Install Referrer / Deep Linking
Relevant Platforms: Android (for attribution)
Relevant Channels: All
Deferred Deep Linking remains the most robust and flexible system for driving measurement and attribution across the Android ecosystem. Functionally, it consists of dynamic URLs which, when embedded in an ad and clicked on by a user, generate a unique ID that is captured by the Google Play Store. If the user does not have this app installed and proceeds to download and open the app, the app’s Mobile Measurement Partner (MMP) requests this “referral content” from the Play store – this includes the unique ID tying back to the specific ad, a click timestamp, and an install timestamp. If the user already has the app installed, the link triggers a custom routing schema that sends the user “deep” into a specific page within the app, as opposed to the launch screen.
Your MMP will be able to assign channel credit based on whatever logic is set up. This system provides direct first-party attribution data to support ad optimization, but also allows existing customers to have a more seamless experience similar to web browsing. The data captured by this architecture is natively integrated into your MMPs reporting suite and available for ingest. Ad-attributed in-app actions can be tied back throughout the user’s lifecycle, allowing for clean and comprehensive cohort analyses.
The primary blindspot of deep linking is that it does not provide any conversion event signal that algorithmic channels like Meta and Google need to effectively optimize performance. It is great for attribution, reporting and UX, but will need to be used in tandem with another solution (see below) to power channels.
On iOS, the deeplinking framework can still be applied, in which you set up a schema of destinations within your app and pass relevant users directly to specific content, however the Install Referrer piece does not pass through the App Store and it cannot be used for attribution/reporting, rendering it a nice-to-have UX tool.
SAN / SRN
Relevant Platforms: Android (and Apple Search Ads)
Relevant Channels: All
Self-Attributing/Self-Reporting Networks – the vestiges of the Device ID (“IDFA”/”AAID”) powered system which drove most of digital advertising through the 2010s. While this attribution framework is heavily under-threat for potentially exposing users to a degree of privacy risk, it actually remains the way that most major digital channels are attributed. When a user installs an app, the app’s MMP calls the various SAN channels (Meta, Google, Snap, Twitter, Apple Search) to ask if they have served an impression to, or seen a click from the user’s device within a predefined attribution window (usually 7d for click & 1d for impression). If multiple channels say ‘yes’, the MMP is able to resolve multiple claims of credit according to the set attribution logic. This yields a clean, deterministic datastream of device-attributed events allowing flexible cohort evaluation over a potentially infinite time horizon.
On Android, this functionality is still in-place. Lucky you!
One important nuance on Android specifically for Meta is that if you attribute your Meta traffic using the SAN endpoint, MMPs are only allowed to provide that information in aggregate reporting. That means any event or user-level exports will show Meta-attributed data as ‘Restricted’ and any channel metadata is removed. If you attribute Meta using Install Referrer, you will be able to access user-level attribution data for Meta via your MMP.
On iOS, the rollout of iOS14.5 (discussed in multiple subsequent sections) dictates that the specific user must ‘dual opt-in’ for this process to function. This means the user must specifically enable the sharing of his or her device’s ID both within the newly installed app, and within the app of the advertising network. Dual opt-in rates tend to be low for a number of reasons, including that trust levels towards major tech giants are relatively low. The small stream of dual opt-in users you do get can provide valuable insights for downfunnel conversion and monetization rates, which can be leveraged as proxies when evaluating data from more limited frameworks, such as AdAttributionKit (discussed below), where visibility and granularity is far more restricted.
“Probabilistic”
Relevant Platforms: Android, iOS (but against Apple Terms of Service)
Relevant Channels: All
The attribution framework now referred to as ‘probabilistic attribution’ was, for many years, referred to as “fingerprinting”. Prior to Apple releasing iOS14.5 in 2021, this system was primarily used by advertising channels where no direct click and device information was available – think Podcasts or Connected TV.
When a user is served an ad, the advertiser’s MMP collects a series of metadata including IP address, device name, device type, OS version, and mobile carrier. When the app opens for the first time after install, the MMP generates a corresponding fingerprint ID using the same data. In theory, with a long enough set of attributes, no two devices will have the same set, allowing for a ‘deterministic’ attribution to be made. In practice, factors like the instability of IP addresses force MMPs to use this data to form a probability distribution of all the campaigns that are likely to have driven the install.
For a long time, this method was only used when it was the best option available. Apple’s rollout of iOS14.5 and App Tracking Transparency specifically banned the practice in its terms, stating “you may not derive data from a device for the purpose of uniquely identifying it.” This threatened iOS developers who attempted to circumvent the new restrictive, opt-in based measurement regulations with the possibility of being removed from the App Store.
For reasons unknown, though much speculated, those threats never came to fruition outside a few early, obscure examples. In its wake, the use of probabilistic matching has flourished on iOS, as it circumvents many of the heavily restrictive and obfuscating elements of SKAN/AdKit (discussed below). Most importantly, it allows for the direct device-level tying of marketing/pre-install activity to post-install/in-app activity. With other currently available methods on iOS, this bridge cannot be made, leaving advertisers severely limited in their ability to assess critical things like user quality & LTV by creative, campaign, and even channel.
Increasingly, server to server probabilistic measurement has become more prominent. Most channels have some form of server to server conversion API (CAPI) that allows for sending user details along with a server to server conversion event in an attempt to match ad impressions and clicks with downstream actions without relying on click attribution. This approach avoids on device scrutiny from Apple, and also avoids many of the issues involved with cross platform tracking, since they allow for tracking on user data, as opposed to being strictly limited to device data.
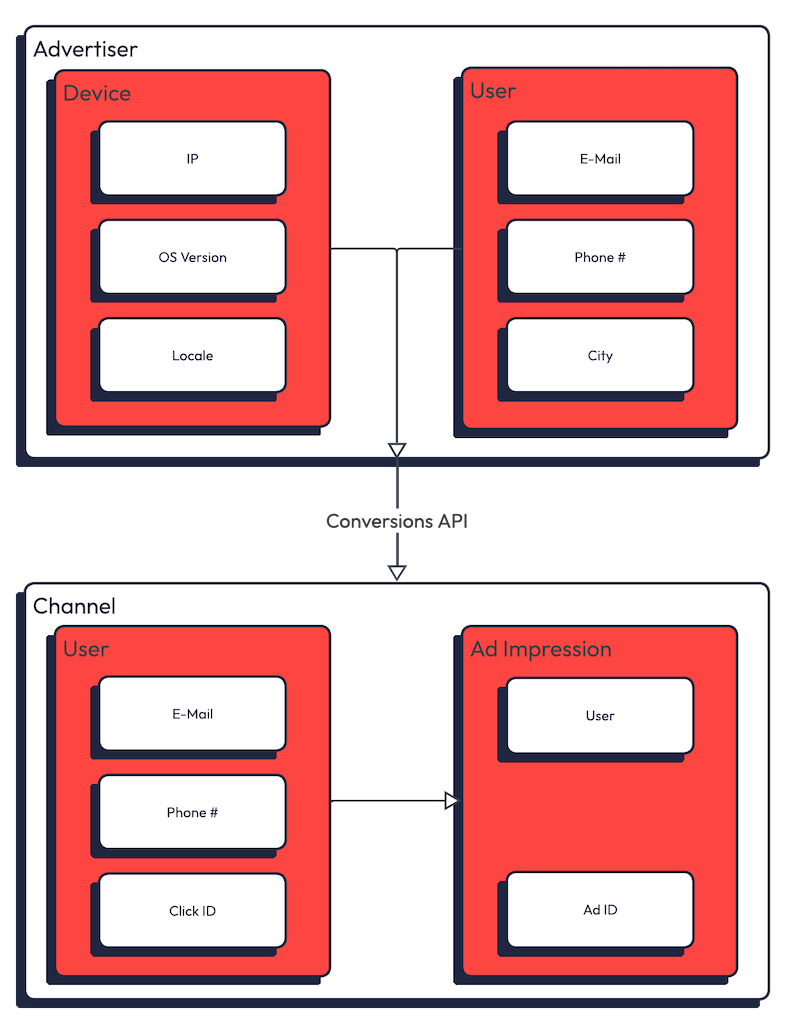
Apple AdAttributionKit (FKA SKAdNetwork)
Relevant Platforms: iOS
Relevant Channels: All (except Apple Search Ads)
Ah, SKAdNetwork. Dead before we really knew you.
The European ‘Digital Markets Act’, which went into effect in May, forced Apple to support third-party standalone app stores, some of which are already going live this summer. Apple’s ‘SKAN’ only functioned within the App Store, leaving Apple no method of attributing traffic through these alternative stores (other than the technically forbidden ‘Probabilistic’).
‘AdKit’, announced in early June, is at this point essentially a rebranding of SKAN 4.0 that now supports these third-party app stores.
Truthfully, explaining the obtuse mechanics and nuances of Apple’s “privacy-centric” attribution framework would double the length of this article. Our co-founder Kevin Young wrote an in-depth perspective on how the functionality impacts ad strategy and measurement not long ago.
In short, AdKit allows iOS advertisers to provide a limited conversion signal to channels constrained within a 48hr window post-install. The complexity and efficacy of this signal are drastically reduced compared to the prior world of SANs and limitless deterministic device-level signal. Furthermore, because all reporting data is blinded and aggregated at the campaign-level, critical assessments of things like creative-level performance and post-48hr user value are not possible.
At the time of writing, adoption of the latest SKAN 4.0 features, most notably the two additional “coarse grain” postbacks, has been minimal from ad platforms. Meta’s Aggregated Event Measurement framework (‘AEM’) rolled out support for app campaigns in Fall 2023. This offers multiple benefits over SKAN including near realtime conversion feedback and no 64-bit conversion limit. Recent indications have been that this is performing well, though clearly in violation of Apple’s privacy regulations, so its path forward is unknown.
Google Privacy Sandbox
Relevant Platforms: Android
Relevant Channels: All
The rollout of Privacy Sandbox, Google’s much-delayed solution to third-party device ID deprecation, seems imminent, though a specific date is not yet known. It is an umbrella term for a suite of tools that will allow advertisers to get critical optimization signal and reporting data to channels and tech partners, while looking to avoid most of the headache that Apple created with its deployment of iOS14.5.
The ‘Install Referrer’ method discussed at the top will still function for Android, so we expect most advertisers will continue to leverage that for reporting, but not for channel signal. SAN channels will need a non-Device-ID-related way to drive delivery optimization. Google accomplishes this in the following way:
Attribution Reporting for Android (ARA) is the portion of the Privacy Sandbox that essentially maps to AdKit and performs a largely similar function. It comes with a set of event level and aggregate reporting APIs that should allow for a bit more insight and granularity than AdKit provides. It consists of three conversion event postback windows: 0-48 hours; 49-168 hours (D7); and a custom third window that can be open up to 30 days post-install. Each postback is sent one hour after the window closes. The ARA conversion data is aggregated at the campaign level and will primarily be used for ad network signal.
Protected Audience API (yes, Google is calling it PAAPI) is a novel concept where users are registered on device as being part of a potential custom targeting segment, allowing ad publishers to take that information into account during ad selection without building in-platform user segments. This audience represents a group of users on a specific device that share certain characteristics or interests relevant to an advertiser. Custom audience information is stored entirely on the user’s device, not shared with app developers or advertisers directly. Through an SDK, advertisers will be able to create custom logic for how these audiences are defined (eg. users who take a certain action, but not another). The specific use cases of this tool are yet to be determined.
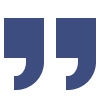
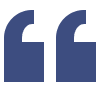
So What?
Each of these different attribution sources has its own strengths and weaknesses. Channels can attribute view-through conversions and provide superior cross-platform attribution via probabilistic matching on user identity, but they are prone to claiming all users they’ve seen, even if those users ultimately converted due to ads on another platform. SKAN can provide solid install attribution at a campaign level, but it is ineffective for down-funnel user actions after the initial conversion window. Deep linking and referrer data provide excellent click attribution on platforms that support it, but this isn’t possible on iOS. So, how do we leverage the strengths of each attribution methodology while avoiding the weaknesses?
Mobile Measurement Partners (MMPs) play an important role in consolidating these disparate attribution sources. However, the real challenge—and opportunity—lies in leveraging this consolidated data alongside more sophisticated marketing analyses, such as incrementality testing and media mix modeling (MMM), and direct feedback from How Did You Hear About Us (HDYHAU) surveys. It is no longer sufficient to rely on a single attribution methodology. A strategy that incorporates each of these approaches when and where they are strongest is the only way to gain clarity on real-world marketing performance.
To truly maximize marketing effectiveness, it’s essential to adopt a holistic approach that blends the strengths of various attribution methods, complemented by advanced analytical techniques and direct consumer feedback. This multifaceted strategy ensures a more accurate understanding of marketing impact, driving better decision-making and optimized marketing investments.
Headlight’s team of experts are offering a free strategic Audit Session across Growth, Creative & Analytics – a unique opportunity for brands to learn more about how to scale spend with incrementality in mind.