Quick Read
- At Headlight we’re always evaluating emergent tools that could provide unique and actionable marketing measurement insights for our partners
- Randomized controlled trials are one of the cleanest and most statistically sound ways to establish incrementality, however in-practice they are often impractical to run
- Meta’s GeoLift, an open-source tool for facilitating cleaner test and control populations, has been gaining traction as a tool for designing Match Market tests
- In the article below we outline why GeoLift’s synthetic geographic regions support more effective incrementality studies and smarter marketing decision-making, as well as some key caveats to take note of
Understanding Incrementality in Digital Marketing
Understanding the true impact of your advertising spend is essential. Incrementality is a loaded term that can be construed in many ways, but at its heart, it is about determining the causal effect of marketing spend. By measuring how marketing activities causally impact desired outcomes, incrementality testing helps us make informed decisions about our investments, helps the advertiser understand the impact of marketing efforts, and helps data teams validate the accuracy of their models.
Determining the causal effect of interventions isn’t unique to marketing, and there is a whole branch of statistics dedicated to it: causal inference. The most reliable way to establish causality is through a randomized controlled trial (RCT). In such experiments, participants are randomly assigned to either a treatment group that receives the intervention or a control group that does not. This randomization helps ensure that any differences observed between the groups are due to the intervention itself.
Challenges with Traditional RCTs in Digital Marketing
However, in the realm of digital marketing, conducting true RCTs is often impractical. Advertisers lack the ability to create a perfect control group because they do not have control over who sees their ads at an individual user level. Ad display is a process controlled by media publishers, and only they have the granularity needed to produce a proper holdout group. For example, Meta’s conversion lift studies accomplish this by showing a placeholder ad to a random selection of users that would have otherwise seen the actual ad.
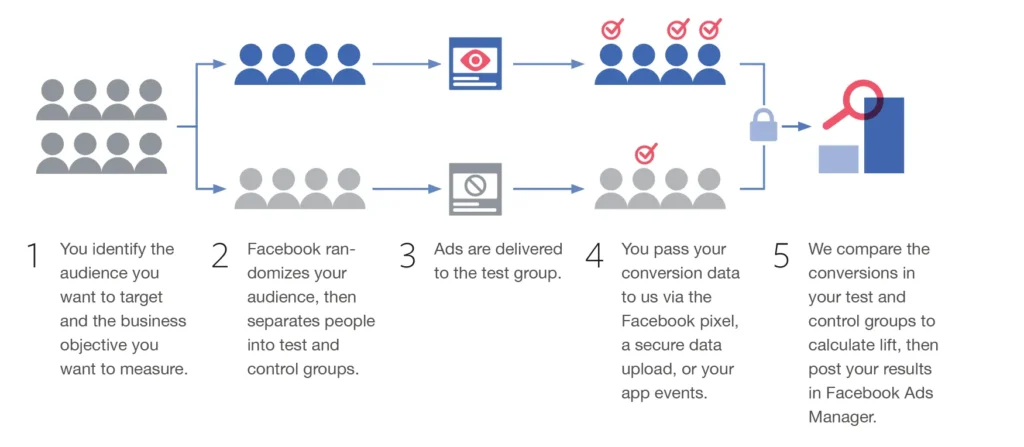
There are a few key drawbacks to channel-run conversion lift studies, though. First off, it is a bit of a black box, and it’s reasonable to be skeptical of an experiment run by the entity you are evaluating (the classic ‘grading your own homework’ scenario). Secondly, we will inherently be dependent on the channel’s conversion reporting for comparing the treatment and control groups. Oftentimes, incrementality comes into question because of assumptions around attribution and instrumentation issues, so in an ideal world, we’d isolate attribution and instrumentation as a source of error in any testing we do. If signal and instrumentation issues are part of the root cause impacting incrementality, running a study solely relying on that framework for test and control will teach us nothing.
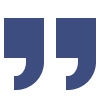
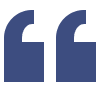
Modeling the Counterfactual with GeoLift
Given these limitations, we have to turn to alternative methods for determining what would have happened if the marketing actions weren’t taken—a scenario known as the counterfactual. By modeling the counterfactual, we can compare it to actual outcomes and assess the incremental impact of our marketing efforts. Several techniques exist to model the counterfactual, each with its own strengths and weaknesses. One powerful approach is the use of synthetic control methods, and this is where GeoLift comes into play.
GeoLift is an open-source library developed by Meta, designed to help perform match market incrementality testing using synthetic geographic regions as experimental units. Geo-based match market testing is not a new concept, but it can be difficult to execute. A naive approach, where we pick two regions and make changes in one while not changing the other, is susceptible to numerous confounding factors, the biggest of which is the assumption of parallel trends. Essentially, in order to effectively compare two regions, we need to strongly believe that those two regions would have behaved nearly identically if no actions had been taken. This can be very difficult to achieve, particularly for earlier stage businesses who may have a wide spectrum in geographic media weight, awareness, and product stickiness (eg. any product benefitting from localized compounding network effects).
How Synthetic Control Works
Synthetic control methods work by creating a weighted combination of control regions (that did not receive treatment) to construct a synthetic version of the treated region. Think of synthetic control as a way to create a “virtual twin” of the treated region by blending other regions that, in aggregate, historically performed very similarly to the treated market. The advantage of this approach is that it abstracts away the complexity of evaluating conversion rates, demographics, and other factors that might influence performance, and helps address issues like spillover effects. Instead, we trust that if historically the synthetic control behaved like the treatment region, then it is reasonable to assume it would have continued to do so during the test period, allowing us to measure the true impact of the campaign.
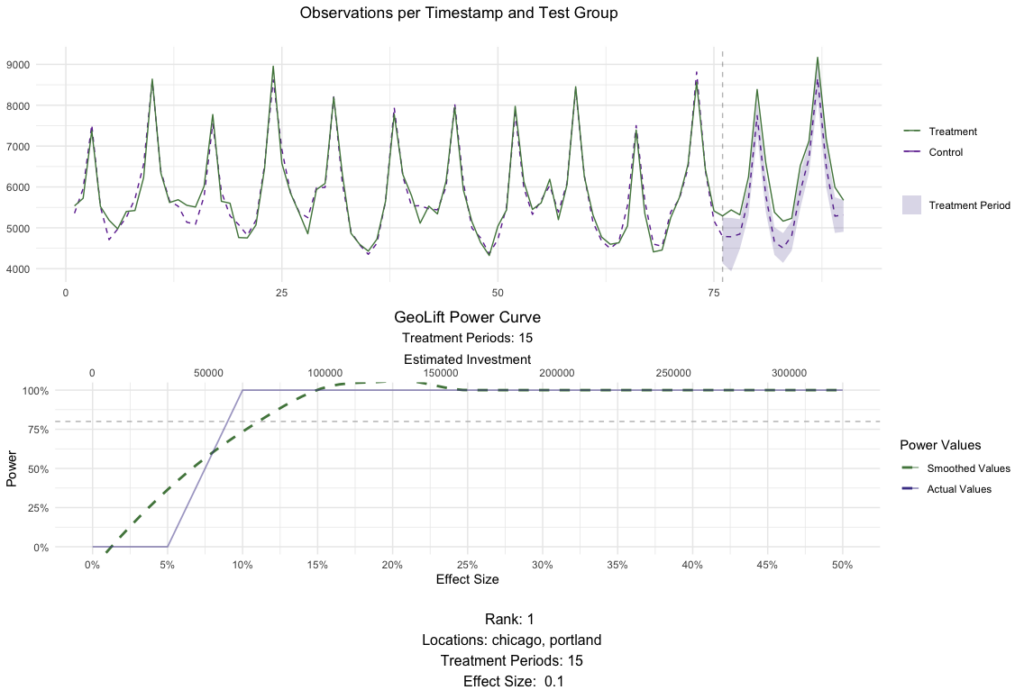
Once the lift—the additional conversions or sales attributed to the marketing effort—is determined using GeoLift, we can gain a clear indication of the impact of our marketing actions. Whether we are adding or removing spend, comparing the treatment and control allows us to understand lift. What this means to us depends greatly on the structure of the test, but it can answer questions like: What is our cost per incremental conversion (CPIC)? What is the impact of this channel in isolation or in combination with other channels? Or, with a multi-cell test, which strategy has a larger impact?
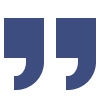
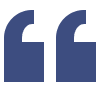
Caveats and Considerations with GeoLift
GeoLift does come with some caveats, however. It requires a significant amount of high-quality data, as it relies on building an accurate historical model. Generally tests will need to run at least three or four weeks, and will require six to eight times that much data in advance at a minimum for running power analysis simulations. Additionally, while the abstract mathematical approach helps sidestep the complexity of directly comparing market attributes, it also makes it difficult to reason about how the control markets are selected. This lack of transparency can make some people uncomfortable with trusting the results.
Integrating GeoLift into Your Measurement Strategy
Incorporating GeoLift into our overall measurement methodology enhances our ability to make data-driven decisions. By accurately estimating the causal impact of our marketing efforts, we can allocate budgets more effectively, optimize campaigns, and demonstrate the value of marketing activities to stakeholders. GeoLift can also be combined with other methods like media mix modeling (MMM) or attribution analysis to create a more comprehensive view of marketing performance. While no method is without limitations, tools like GeoLift offer a practical solution for overcoming the challenges of traditional incrementality testing in digital marketing. By leveraging geographic data and synthetic control methods, we gain deeper insights into the true effectiveness of our campaigns.
Conclusion
If you’re interested in applying incrementality testing with GeoLift or want to enhance your campaign measurement strategy, our team at Headlight can help you get started. Reach out to learn more.
Headlight’s team of experts are offering a free strategic Audit Session across Growth, Creative & Analytics – a unique opportunity for brands to learn more about how to scale spend with incrementality in mind.